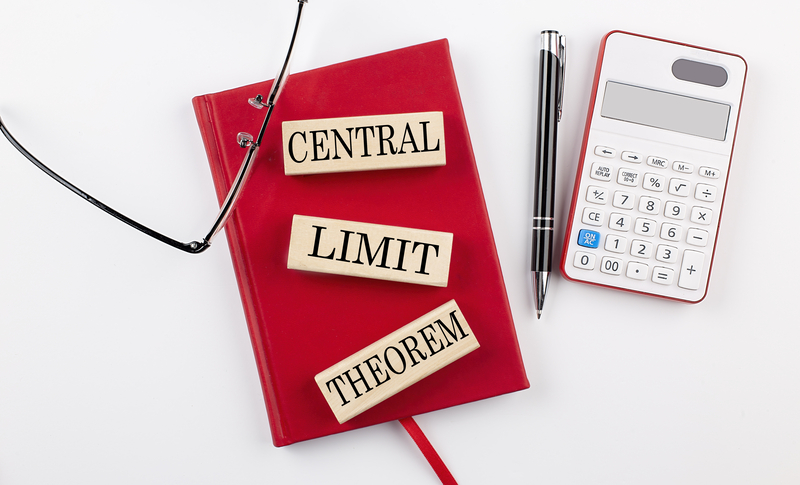
In the dynamic landscape of modern business, data-driven decision-making reigns supreme. As organizations seek to harness the power of information for strategic advantage, a pivotal tool emerges: the Central Limit Theorem (CLT). This theorem transcends data’s inherent complexity by enabling businesses to transform raw information into actionable insights. With the ability to uncover hidden trends and patterns, CLT serves as a guiding light for better decision-making. This article embarks on a journey to unravel the potential of CLT, delving into its mechanics and unveiling strategies that empower businesses to make informed choices that shape their success.
Understanding the Central Limit Theorem: Laying the Statistical Foundation
The Central Limit Theorem stands as a beacon of statistical insight, unraveling data’s intricacies. By embracing CLT‘s concept, data transforms into a symphony of normal distribution—a format suitable for further analysis. CLT’s elegance lies in its ability to take complex data and unlock its potential for informed decision-making—a fundamental principle vital to businesses seeking clarity amid the data-driven age.
CLT is a cornerstone of statistics, unraveling the mystery of data distribution. It asserts that as sample sizes grow, the distribution of sample means approximates a normal distribution—irrespective of the original data’s distribution. This phenomenon enables analysts to infer population characteristics with greater accuracy, even when dealing with non-normally distributed data. CLT empowers decision-makers by transforming diverse data sources into a common language, facilitating more robust and reliable insights for strategic choices.
Leveraging Sample Size for Precision
Amid the intricate dance of data, sample size emerges as a critical partner to CLT. Larger sample sizes amplify CLT’s effectiveness, minimizing the impact of outliers and random variations. This alliance enhances the precision of estimations, enabling businesses to derive more accurate insights about population parameters. By optimizing sample sizes, organizations not only elevate the reliability of their data-driven decisions but also fortify the foundation on which strategic choices rest.
Enhancing Decision-Making with Confidence Intervals
Confidence intervals, derived from CLT, serve as a compass in the sea of data uncertainty. These intervals provide a range within which population parameters are likely to fall, bolstering the confidence of decision-makers. By incorporating the margin of error, organizations gain a clearer perspective on the reliability of their insights. Confidence intervals transform data from a mere collection of numbers into a strategic tool that empowers businesses to make informed and impactful choices.
Mitigating Uncertainty through Hypothesis Testing
Hypothesis testing acts as a compass in the fog of uncertainty. By leveraging CLT’s power, businesses validate assumptions and draw conclusions about population parameters. This process instills evidence-based confidence in decisions. Hypothesis testing transforms raw data into actionable insights, enabling organizations to steer clear of assumptions and navigate the realm of facts. It empowers businesses to embrace uncertainty, transforming it into an opportunity for informed and strategic decision-making.
Navigating Data’s Pathway with Central Limit Theorem
In the symphony of data, the Central Limit Theorem orchestrates harmony. By distilling complexity into a powerful toolkit, it transforms businesses into data-driven decision architects. Harnessing the theorem’s essence, organizations traverse uncertainty with confidence, sculpting a future shaped by precise insights and strategic mastery.
Comments are disabled for this post.